new research supports integrated physical and mental health care
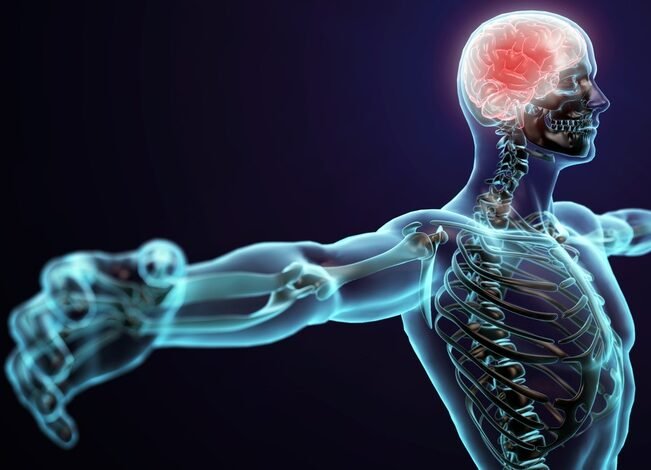
The French philosopher René Descartes is often credited with discovering the mind-body problem; a dogma with long-standing implications on psychiatry. Descartes introduced notions that the mind and body are separate entities, albeit later acknowledging that there may indeed be a link via the brain (specifically, the pineal gland). Resultantly, modern psychiatry largely neglected influences of the peripheral body on mental health, with biological psychiatric research often taking a more brain-centric role.
But is this truly the case? Is one’s mental state simply a product of our brain function? The evolving field of ‘psychoneuroimmunology’ (PNI) for example, begs to differ. As the portmanteau might have suggested, PNI is a hybrid research area – intersecting psychiatry, neuroscience, and immunology. Importantly, PNI research has shown time and time again that the peripheral body has distal effects on mental health. Previous blog posts by The Mental Elf have also discussed findings supporting this idea (Corsi-Zuelli, F., 2023; Foley, É., 2023; Parker, N. and Andreassen, O., 2023).
To further our efforts towards ending the enduring influence of Cartesian dualism in psychiatry, this blog discusses a recent study by Tian et al., (2023) published in JAMA Psychiatry. This paper compares brain and peripheral bodily system alterations across psychiatric disorders to healthy (control) individuals. The article addresses a timely question in psychiatry, both from a therapeutic and pathophysiological standpoint – what is the importance of physical comorbidities and physical health in our evaluation of psychiatric disorders/mental health?
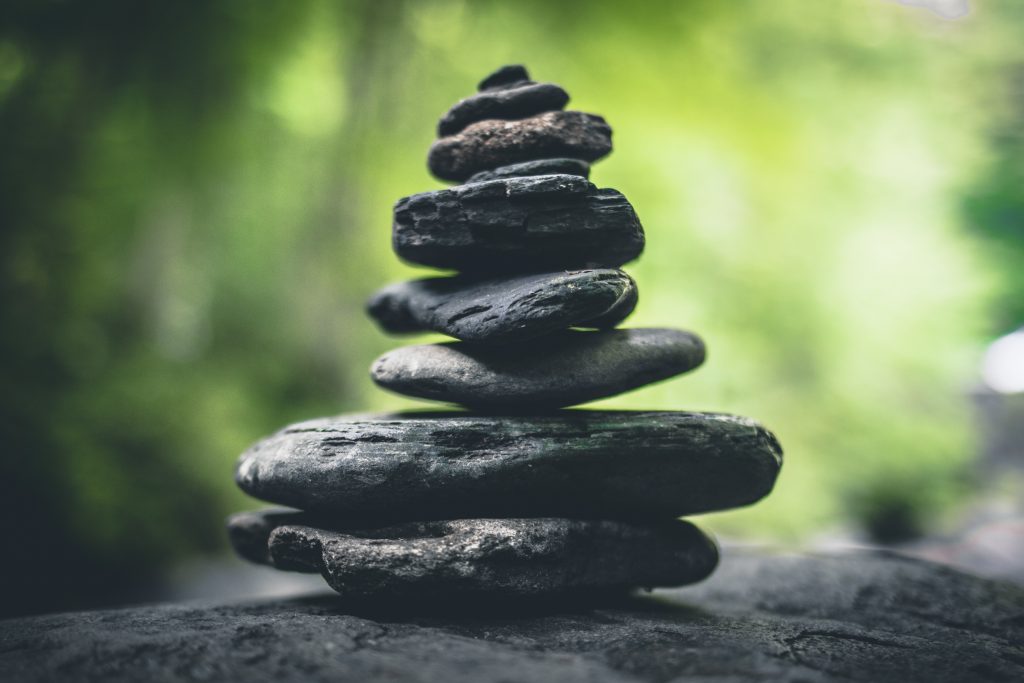
The Cartesian notion of the mind and body being separate entities has negatively influenced our view of mental vs physical health. In reality, our mind and body work hand-in-hand to determine our overall health and wellbeing.
Methods
Participants and data source(s)
This study leveraged seven publicly available cross-sectional datasets to integrate brain imaging data (structural and diffusion-weighted magnetic resonance imaging [MRI]) with physical and physiological data. Physical and physiological assessments were sourced from 175, 944 individuals participating in the UK Biobank. Participants were further split into two groups: Controls without a neuropsychiatric diagnosis (N=87,420) and those diagnosed with a neuropsychiatric disorder (N=85,748; schizophrenia, bipolar disorder, depression, generalized anxiety disorder, dementia). People who were comorbid for any of the evaluated psychiatric disorders were excluded.
Imaging-Derived Phenotypes (IDPs), or brain regions of interest, examined in the current study included proxies of:
- Regional gray matter (GM) health (NIDP=76; of T1-weighted MRI origin)
e. cortical thickness, and cortical as well as subcortical GM volume. - Brain white matter (WM) (NIDP=54; of diffusion-MRI origin)
e. tract-specific measures of WM microstructure such as fractional anisotropy and mean diffusivity.
Relevant peripheral physical and physiological measures (73 in total) were selected and grouped into seven bodily systems, i.e. pulmonary, musculoskeletal, kidney, metabolic, hepatic, cardiovascular, and immune systems. All input (brain, blood and urine biomarkers, physiological measures) was quality controlled and harmonized for analytic use.
Analytic approach
The study presents a two-step analytic approach to evaluate brain-body health. The initial step involved ‘normative modelling’ i.e., building reference ranges for the various brain and body variables by using measures derived from controls without a neuropsychiatric diagnosis. The resultant reference ranges were then used to estimate deviation scores – from ‘normal’ range – for individuals diagnosed with a neuropsychiatric disorder.
Secondly, an organ health score (OHS) was estimated for each bodily system including the brain, i.e. one for brain GM and one for brain WM, per individual. The OHS is a weighted sum of deviation scores across all organ/system-specific traits. Seven OHS were ultimately generated for each individual (one for each of the bodily systems/organs examined), with an additional eight organ-specific health scores, i.e. overall body health score, computed based on patients with chronic diseases affecting multiple bodily systems. Lower organ-specific health scores denote poorer ‘health’.
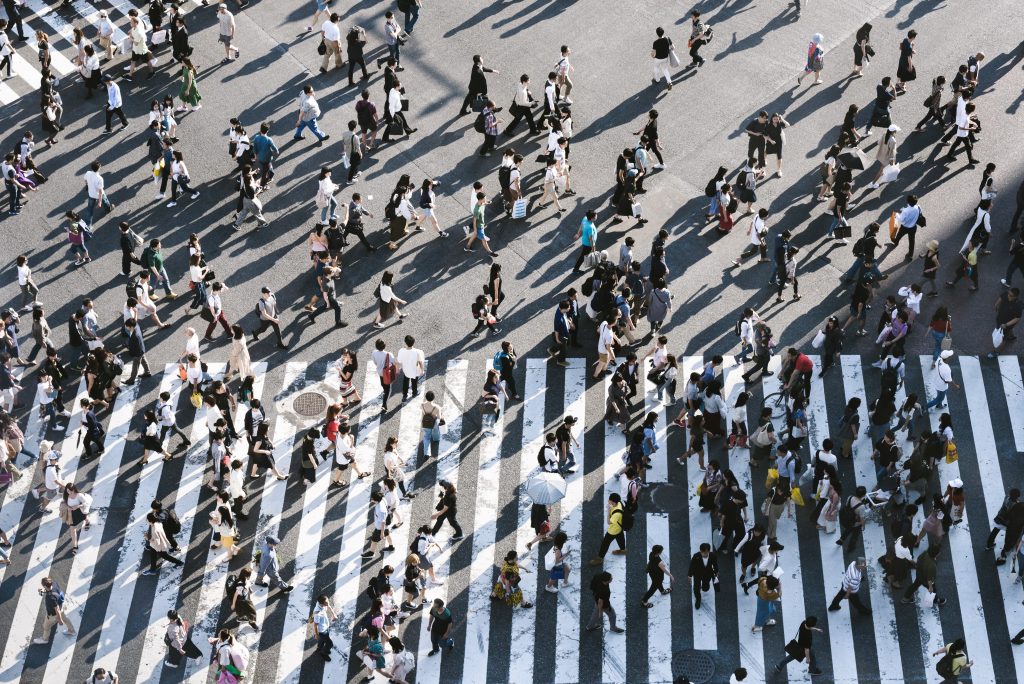
To understand the value of physical health in mental health, this study evaluated ‘health’ within brain and peripheral bodily systems of five neuropsychiatric disorders vs individuals without any diagnosis.
Results
The authors evaluated brain-body health under two streams of stratification:
- Organ/system – differences in OHS were compared between neuropsychiatric disorders and control group.
- Diagnosis – the eight OHS were compared within the selected neuropsychiatric disorders.
This evaluation strategy aimed to reveal two levels of information:
- Which brain and/or body health scores were markedly different across the different neuropsychiatric disorders compared to controls (if any), and
- Which brain and/or body health score is perhaps best at discriminating neuropsychiatric diagnoses.
On average, all organ-specific health scores were significantly lower in those with a neuropsychiatric disorder diagnosis compared to age- and sex-matched individuals without a diagnosis. Notably, metabolic, hepatic, immune and renal health were most deviated from normative reference ranges for schizophrenia, bipolar disorder, generalized anxiety disorder, and depression. In other words, poor body health (peripheral systems) was a more pronounced ‘marker’ of neuropsychiatric disorders – or mental ill health – than brain changes when compared against individuals without a neuropsychiatric diagnosis.
Within the group of patients, brain health scores were more accurate discriminators of neuropsychiatric diagnoses than body health scores. Evaluation of OHS by diagnostic status revealed that brain health (GM and WM) was poorest in individuals with schizophrenia. Interestingly, this was only marginally poorer in bipolar disorder, depression, and generalised anxiety relative to controls.
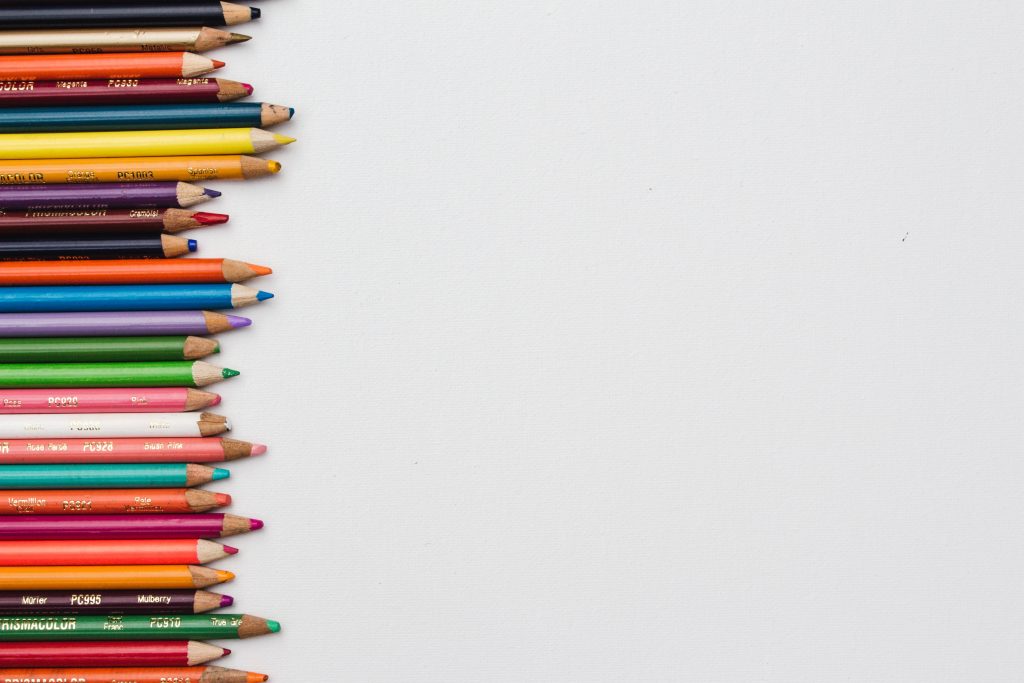
Poor physical health was a better ‘marker’ of mental ill health than brain changes when comparing cases to controls, but brain health was superior when distinguishing between neuropsychiatric diagnoses.
Conclusions
The findings from this investigation unequivocally lend support to the role of physical health in mental health. The authors further concluded that:
Poor body health and function may be important illness manifestations that require treatment in patients.
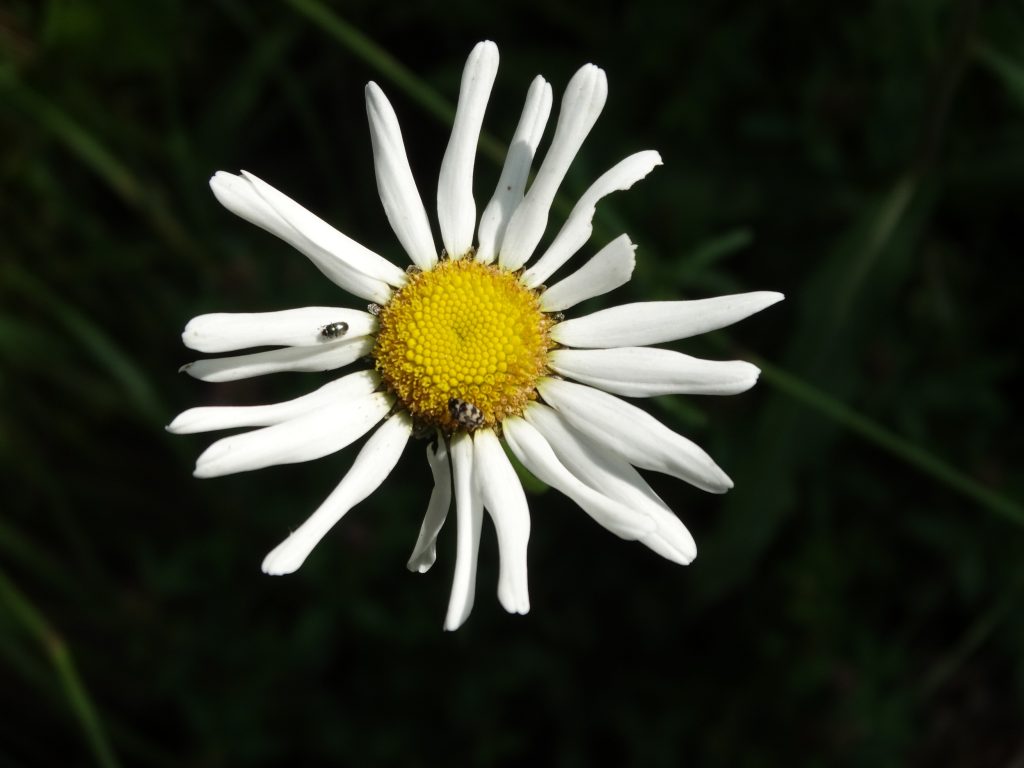
Poor physical health is an important determinant of mental health, as is brain health specifically.
Strengths and limitations
Strengths
A novel analytic component of the study relates to the generation and usage of normative reference ranges. Since the authors leveraged population/community-based datasets, it was perhaps tricky to establish a clinically ‘healthy’ subgroup for comparison. Instead, the authors circumvented this issue by building a normative distributional curve, pooling individuals without neuropsychiatric disorders across datasets for brain phenotype evaluation, and similarly for peripheral bodily systems using the UK Biobank dataset. In this context, the approach i.e. ‘normative modelling’ is an inventive statistical solution as it:
- Permits individual-level inference, and
- Is a flexible framework devoid of rigid assumptions e.g. requiring a consistent biological signature across all individuals to establish an ‘average participant’ as a comparator (Marquand et al., 2019).
Normative modelling is akin to building a ‘standard’ benchmark to examine individual-level deviation relative to the population. A current example in clinical practice would be the paediatric growth chart used to map development of children as a function of age.
Limitations
Quoting the authors:
It is also important to acknowledge biases inherent to the cohorts studied. UK Biobank predominantly comprises individuals of ‘White British’ ancestry.
In the present study, all races/ethnicities (or ancestries) were included to make up the analytic sample. Although some ethnic populations may be less represented across cohorts, the inclusion of the entire population in the first place is a commendable effort by the authors as it would be a more realistic representation of true population. Further work is inevitably needed to test robustness of the present findings in individuals of various races/ethnicities or ancestries. Similarly, sociodemographic influences e.g. socioeconomic status, psychosocial stressors and lifestyle habits are known to affect physical and mental health. It would have been interesting to examine disparities in OHS and normative sampling when accounting for these as part of sensitivity analyses.
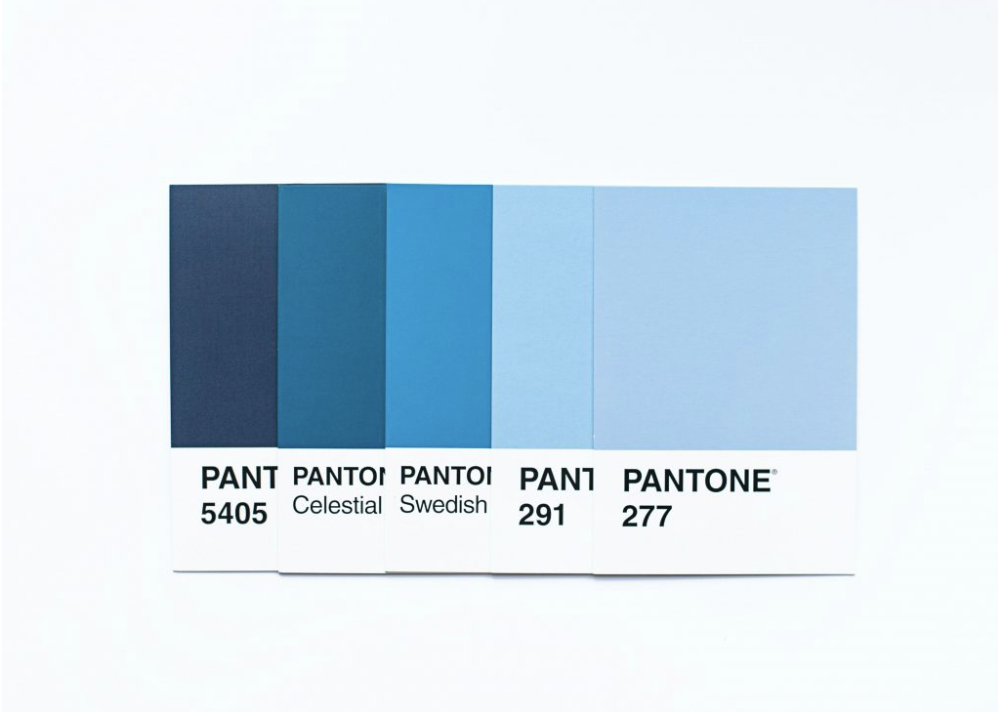
Achieving this is a big feat for psychiatric research – but the added value of racial, ethnic and ancestral diversity in study sampling is crucial for the generalisability of findings.
Implications for practice
Employing organ health scores to support clinical decision making in psychiatric practice is perhaps a little premature. However, this paper has irrefutably made a compelling argument for the role of peripheral systems in mental health evaluation as well as management. Realistically, a metric like the organ health score would require further evolution, evaluation and standardisation for psychiatry-specific use. One example of a metric that was first demonstrated through research are polygenic risk scores – an estimate of the degree to which an individual is at risk of common illnesses owing to their genetic makeup (Wray et al., 2021). It could be thought of as an analogous measure to the organ health score i.e. ‘genetically-derived health score’ for trait or phenotype. In psychiatric research, these genetic risk scores have additionally been evolved to also account for comorbid peripheral disorders e.g. cardiovascular disease in PTSD (Seligowski et al., 2022) to improve clinical utility of such metrics. You can read more about polygenic risk scores in these other Mental Elf blogs (Hagenberg, 2024; Palmer, 2023; Parker & Andreassen, 2023).
In relation to therapeutic management, cardiometabolic, immune and hepatic comorbidities especially warrant consideration. Metabolic disorders, chronic stress and substance dependency (e.g. excessive drinking) are known to be heightened in those with psychiatric disorders. It is important to understand how poorer peripheral health could impact efficacy of psychotropics. The authors accurately noted that several markers of peripheral system health are indeed being readily assayed in primary care settings e.g. lipid panel, blood count (differentials). These could be similarly leveraged and evolved to:
- Investigate their association with other bodily markers and
- Identify disorder-specific multiomic signatures, akin to recent demonstration of this using body mass index (Watanabe et al., 2023).
The same can perhaps be applied to normative modelling. Still a long way from clinical uptake, but new research findings are beginning to explore utility of this framework in psychiatric subtyping (Wong et al., 2023; Shao et al., 2023).
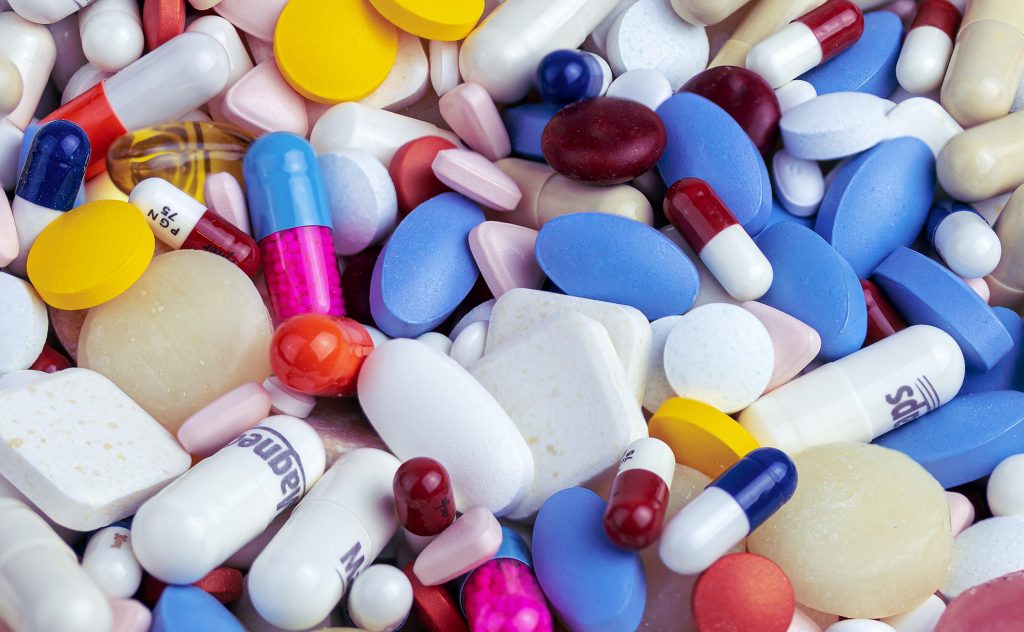
Parallel efforts could be directed towards unpacking how poorer peripheral health could impact efficacy of psychotropics in psychiatric disorders.
Statement of interests
Athina is thematically interested in a similar area of research presented in the current publication i.e. brain-body interaction, systems biology and multiomics.
Links
Primary paper
Tian YEEvaluation of Brain-Body Health in Individuals With Common Neuropsychiatric Disorders. JAMA Psychiatry. 2023;80(6):567–576. doi:10.1001/jamapsychiatry.2023.0791
Di Biase MA Mosley PE, et al.Other references
Corsi-Zuelli, F. Cellular immune phenotypes of depression: a gateway to precision medicine. The Mental Elf, 29 Jun 2023.
Foley, E. Blood-based inflammatory markers in acute vs chronic schizophrenia. The Mental Elf, 19 May 2023.
Hagenberg, J. Can proteomics improve our prediction of depression remission? The Mental Elf, 2024
Marquand, A. F. et al. Conceptualizing mental disorders as deviations from normative functioning. Mol Psychiatry 24, 1415–1424 (2019).
Palmer, E. Genetic risk for Tourette Syndrome and related conditions. The Mental Elf, 23 Nov 2023.
Parker, N & Andreassen, O. Genetic risk for schizophrenia is associated with changes in heart structure and function. The Mental Elf, 31 July 2023.
Seligowski, A. V., Misganaw, B., Duffy, L. A., Ressler, K. J. & Guffanti, G. Leveraging Large-Scale Genetics of PTSD and Cardiovascular Disease to Demonstrate Robust Shared Risk and Improve Risk Prediction Accuracy. AJP 179, 814–823 (2022).
Shao, J. et al. Capturing the individual deviations from normative models of brain structure for depressive diagnosis and treatment. Biological Psychiatry (2023) doi:10.1016/j.biopsych.2023.08.005.
Watanabe, K. et al. Multiomic signatures of body mass index identify heterogeneous health phenotypes and responses to a lifestyle intervention. Nat Med 29, 996–1008 (2023).
Wong, T. Y. et al. Traumatic stress load and stressor reactivity score associated with accelerated gray matter maturation in youths indexed by normative models. Mol Psychiatry 28, 1137–1145 (2023).
Wray, N. R. et al. From Basic Science to Clinical Application of Polygenic Risk Scores: A Primer. JAMA Psychiatry 78, 101–109 (2021).
Photo credits
Source link
#research #supports #integrated #physical #mental #health #care